Data Ethics: Security versus Integrity?
Elijah van Soldt
When we think about the ethics of data, we tend to centre the discussion around whether or not we should be gathering data in the first place. Questions of whether or not it is responsible or even necessary to gather data are omnipresent and so are the popular answers to those questions: yes, of course it is necessary to gather data. After all, it is in our own best interest to do so. It aids in our security and what do any of us really have to hide, anyway?
Artist and scientist James Bridle (a.o. Hacking Habitat, 2016) turns this on its head: gathering data is not in the benefit of the people, it is always in the data gathering corporation’s benefit. Indeed, let us not forget the old adage: if something is free, you are probably the product. If anyone’s security is being aided, it is the company’s security against bankruptcy. But it’s not merely our phone numbers and e-mail addresses that are being gathered, it is the very fabric of our lives: our behaviour is at stake. Bountiful algorithms measure who you are based on your likes and dislikes and tailor their ads to exactly who it thinks you are and will become as a person. Behavioral biometrics is the newest technology tracking our unconscious behavior, from voice and face recognition until tracking even the most subtle nerve tick.
As many brilliant scholars have written extensively about security versus privacy. I would instead like to draw attention to the matter of security versus bodily and mental integrity. We know our data is being gathered and this is certainly not a reality we need to accept as such, but we must deal with it. We must ask ourselves: who gathers our data, how, and to what end? Furthermore, is my personhood reducible to data and if not, what are the consequences and what can we do about it?
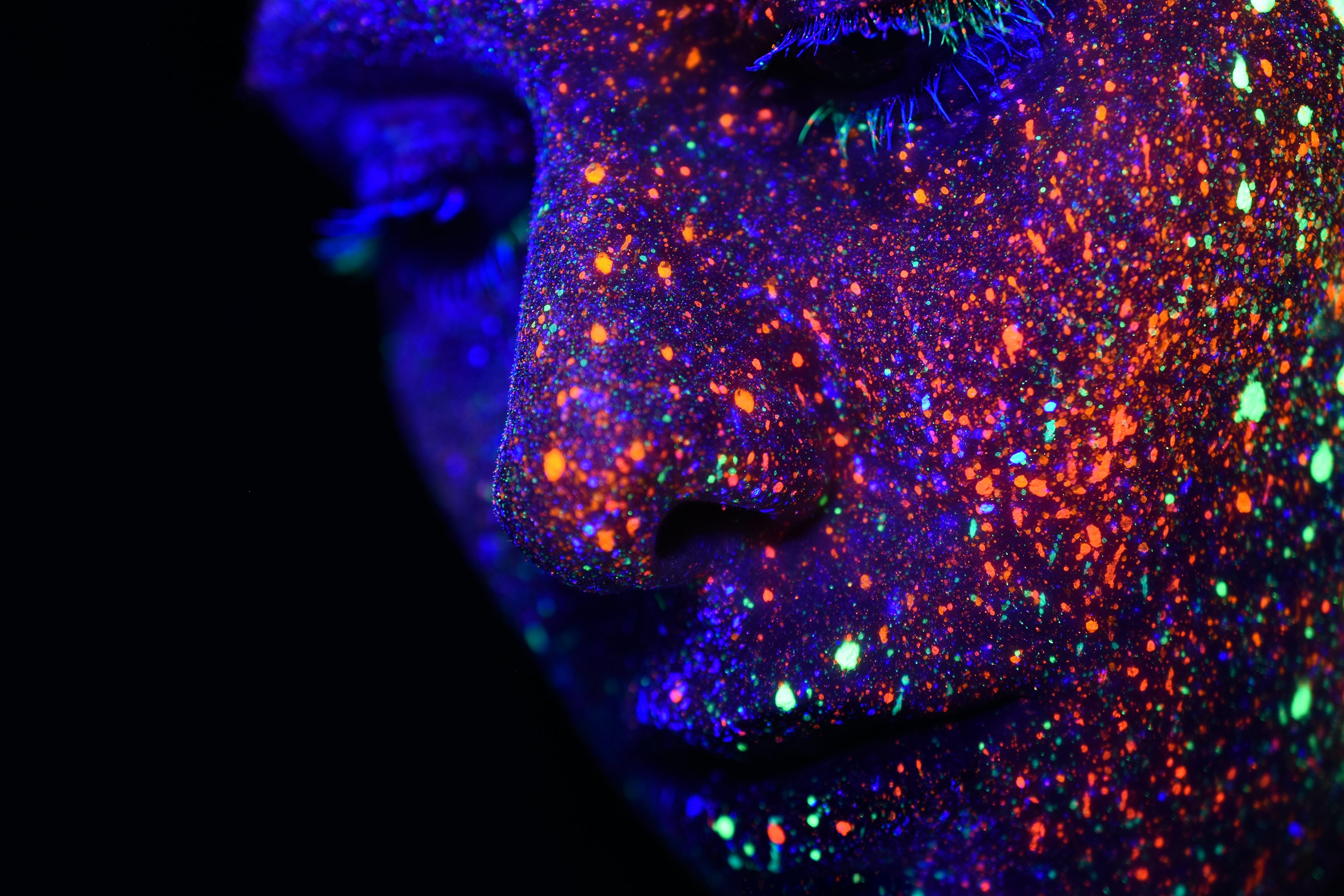
The how and whom of the matter are not insignificant: algorithms and data gathering software are designed by people, and people are fallible and biased creatures. If a developer with implicit racist bias designs an algorithm, chances are it will gather and generate data based on that bias. Twitter, for example, has been guessing people’s genders for years now and simply ‘assigning’ them in Twitter users’ personal data based on their Twitter behaviour. I had not paid much attention to it but needless to say I was quite amused when Twitter gendered me as ‘male’ in spite of me having entered ‘female’ years before. I suppose my transness was powerful enough for the algorithm to recognise.
Amusing though that may be, it is also objectively horrifying. What are the implications of a digital panopticon that simply ‘decides’ what its users are and whose algorithm not only selects biasedly, but also spreads that bias? If we are to be the best cyborgs we can be, how do we make it so that we do not become indentured data?
Fighting a system so broad and so widely used is an impossible feat, so the best thing we can do is to understand it and circumvent it through clever use of the software, or simply by creating entirely new software. Consider, for example, the DuckDuckGo browser, which does not store personal info. We need an awareness of all the ways in which our data is gathered: pictures, location services, face filters on all sorts of apps, Facebook and other massive social media platforms that function as massive personal data bins, YouTube viewing history data, Google services of all kinds, and so on and so forth. Even then, awareness is not enough: these hotspots of data aren’t necessarily secure even when the data is not being actively sold elsewhere. Think of Zoom’s massive 2020 data breach.
Another artist and ‘poet of code’ who understands how algorithms and code work is Joy Buolamwini, who is included in the (IM)POSSIBLE BODIES repertoire. She has started initiatives such as the Safe Face Pledge to bring attention to the harm such technologies can cause and aiming for it to do better. She calls the phenomenon of algorithmic bias ‘the coded gaze’. She points out that these, as I hint at above, can lead to exclusionary experiences and discriminatory practices. What if, because facial recognition software isn’t properly trained to recognise black people, it misidentifies a criminal on account of his skin tone? Worse, what if predictive algorithms in law enforcement start ‘predicting’ the likelihood of a person committing a crime, in the same manner I was ‘predicted’ to be male? How would im- or explicit bias affect that? Especially within the context of the American incarceration system, biased (facial recognition) software making its way into law enforcement is and should be a terrifying thing, and this is not limited to law enforcement or America alone.
A short, comprehensive answer to the problem of maintaining our integrity amongst big data is impossible to give. The long and short version of it is that we must engage, understand and work with data in smart ways. We need to be aware of what parts of ourselves we are so very willing to give away to Snapchat filters and Facebook filter-related social media ‘challenges.’ We need a collective awareness of when we are freely and consciously sharing our data, and when we are simply being played by data gathering ‘games’ or algorithms suggesting everything we’ve ever wanted. Sure, the filter that makes me look like an elder is fun, but who does it benefit more: me having a quick laugh at a silly picture, or the company that just earned itself a perfect overlay of my facial features?